CTCI E-Newsletter
No. 459
APR - JUN / 2019
Technology
Technology
技術分享
Building Smart Engineering with AI--
Engineering Application of Image Recognition Technology
— Jacky Chao, RD Engineer, Research Innovation Center, CTCI Corp., Group Engineering Business
For years, CTCI has been providing the “Most Reliable” engineering service to customers in various aspects including systematic and information-oriented engineering design, procurement and construction management systems, and operating platforms. The efficiency of execution and the capability of interface integration are greatly improved. In 1997, IBM Deep Blue beat the world chess champion, and 20 years later, AlphaGo took down the world GO champion, Ke Jie. Artificial Intelligence (AI), with the advancement of technology, has shown surprising potential and has become a hot topic in the industry. Mastering the advancement of technology, CTCI actively introduces AI in international turn-key projects, which are transformed from systematic- and information-oriented into intelligence-oriented systems. CTCI Research Innovation Center is aggressively pursuing the development goals for intelligent solutions to turn-key projects (iEPC 8 + 1 Digital Initiatives) for an exclusive CTCI digital innovation. AI and image recognition technology can quickly assist digitalization of engineering information, dramatically increase the efficiency of engineering execution, reduce cost, ensure the intelligent turn-key project capability, and, as a result, key strength can be built for a continued leading position in the international engineering market.
Application of Image Recognition Technology
AI has a long history, with its name tracing back to 1956. Since then, “machine learning” has advanced the development of AI. In recent years, the breakthrough development of “deep learning” has boosted the explosive growth and application of AI. Key technologies such as “machine learning” and “deep learning” have made major breakthroughs in image recognition technology. Facebook, Microsoft, Google, Amazon, Apple, and other world-renowned technology companies have all started to develop business opportunities in image recognition. At present, image recognition has been able to perform face recognition, intruder detection, license plate recognition, etc. With the relevant resource investment and technology evolution, more innovative applications shall be developed down the road. Speaking of image recognition, many people may think of face recognition. Besides face recognition, image examination is also widely used in many fields, such as to assist doctors in medical imaging for the diagnosis of diabetic retinopathy, breast cancer metastasis, etc., as well as to help factories identify defective products.
What's the Difference Between Machine Learning and Deep Learning?
“Machine learning” and “deep learning” are two terms closely related to each other but often misunderstood. They actually have a slight difference. Machine learning is part of AI, which focuses more on a specific scope. The basic definition of machine learning is “machine’s learning ability without program guidance.” A large amount of data is input to the computer for an autonomous output of analysis results or implementation. Feature detection function in machine learning requires that programmers instruct the computer to look for information that is “conducive to human decision-making.” Human error factors in the programming process will affect the accuracy of data interpretation. The ability to execute commands through machine learning principles, such as that of Siri, the virtual assistant in Apple's iOS, is also built on voice recognition technology that is capable of processing human language for command execution. The response is getting more and more natural and mature. Deep learning is a branch of machine learning research aimed at bridging the gap between machine learning and the AI. Deep learning (deep neural networks) allows computers to analyze data in order to find the “eigenvalues,” rather than having humans determine the eigenvalues. It looks like computers can “learn” in “depth,” Deep learning uses multi-layer neural networks. Theoretically the more hidden layers there are, the higher degrees of freedom and accuracy it has. In deep learning systems, machines learn how to perform specific tasks by processing large amounts of data and algorithms. When the data is fed into an artificial neural network, the system asks a series of yes-or-no or numerical questions and classifies the data according to the answers obtained. The image recognition system constructed by the principle of deep learning is currently used in the “training” of self-driving cars, face recognition, vehicle recognition, robot development, etc. The difference between machine learning and deep learning is explained with the example of dog identification. In terms of machine learning, it is expected to have a set of data containing all features of dogs, such as shape, color, pattern, etc., the more varieties the better. Then, with human knowledge, some features are selected from the data and a model is trained by the selected data for the identification of dogs. In the aspect of deep learning, the process of feature selection by human knowledge is abandoned, instead, a multi-layer structure neural network learns by itself about how to perform feature extraction from a large amount of data. In other words, the features of dogs are established according to the data you gave to the model, and the model itself learns the differences by features extracted from dogs.
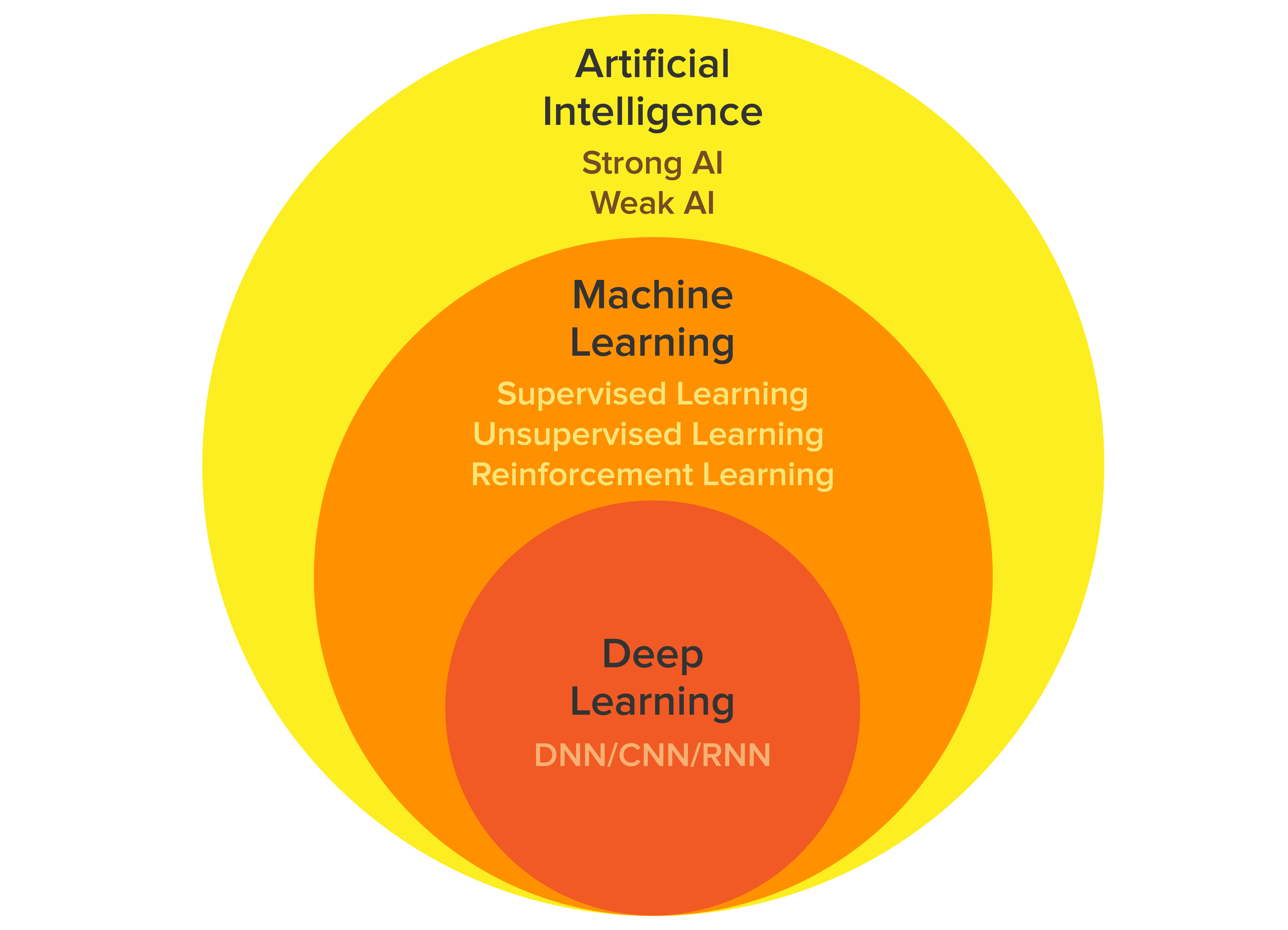
Connections between AI, machine learning and deep learning
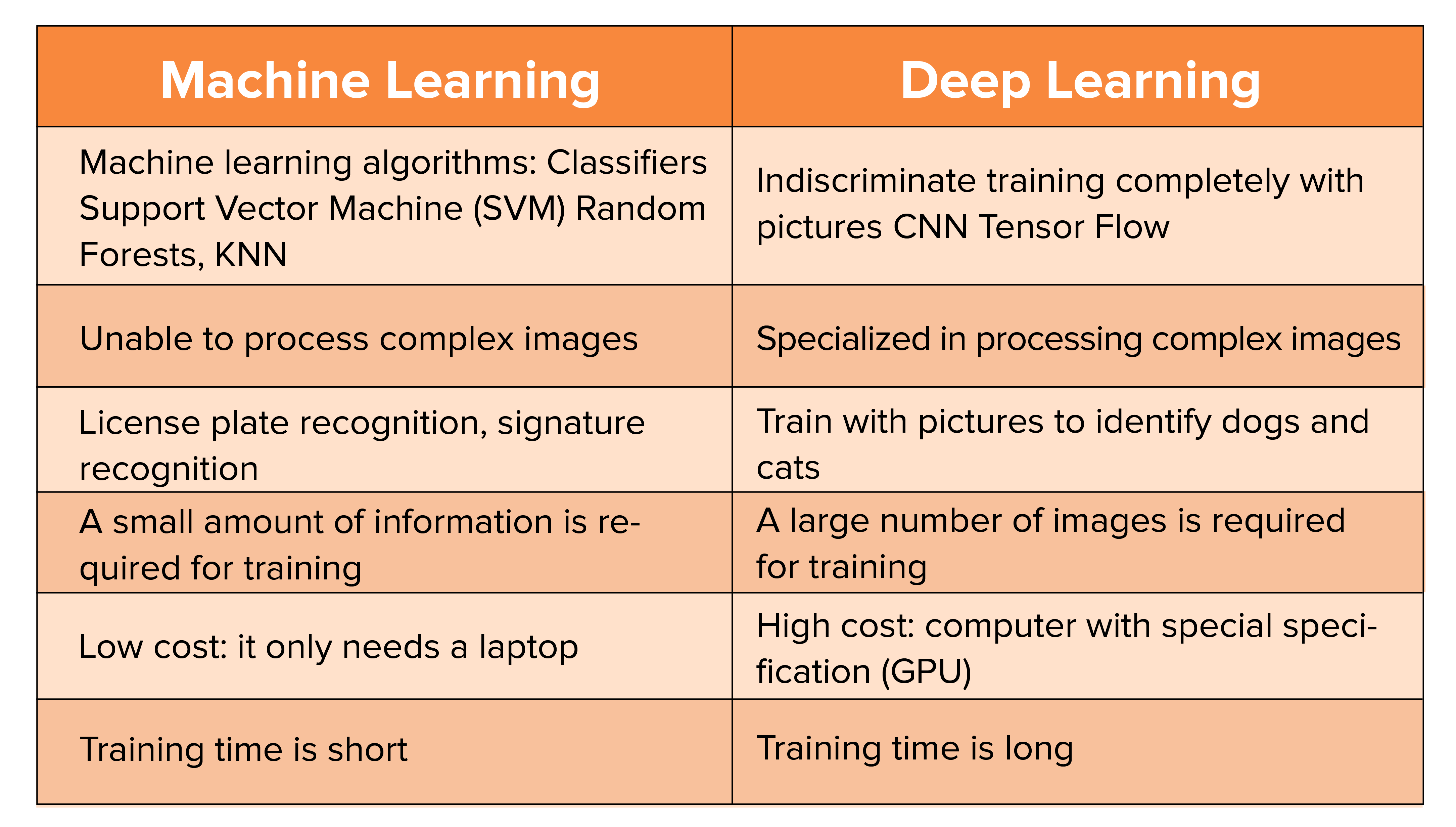
Machine learning versus deep learning
CTCI Introduces AI and Image Recognition Technology to Digitalize Engineering Information
To realize Intelligent turn-key project and to build up core technology capability, CTCI Research Innovation Center has proposed the development goals for intelligent solutions to turn-key project, together with the digitalizing renovation (iEPC 8 + 1 Digital Initiatives). CTCI’s system platform shall be integrated with the latest technology in the market, building a digital engineering exclusively for innovation of CTCI, so that a complete solution can be available to the entire CTCI. The first step of digital engineering is to digitize engineering information. In the Data Discovery and Design Collaboration within the iEPC 8 + 1 Digital Initiatives blueprint, research projects of AI and image recognition technologies shall facilitate the digitization of engineering information. We bring in natural language processing technologies, such as interpretation of various engineering specification and standard to assist and guide engineers by AI. Engineering design standards, as many as 6000, can be quickly located and confirmed as a basis of the engineering quality inspection. Thus, not only work efficiency is improved, mistakes are reduced, but also the quality of engineering design is ensured without increasing cost. In addition, we also bring in image recognition and deep learning technology to help engineers quickly interpret engineering drawings, extract design information from engineering drawings, and complete preliminary design work, so that we have more time to concentrate on high-output design work. Take plant layout as an example, all the equipment and instruments in a plant need to be properly configured within the limited perimeter of the plant. A plant layout should first meet the requirements of laws and regulations, the owner's operating procedure and practices, and then consider the operability, safety, and economics of the relative location of the equipment. The plant layout determines the length of the pipeline and cable channel, which not only affects the cost of the plant construction but also plays an important role in winning the bid. The price for good plant layout is time and manpower. Is there a solution to keep all the benefits without pain? Of course. CTCI has introduced evolutionary computing technology to assist engineers in optimizing plant configuration. Through the expertise and experience of engineers as well as the powerful computing and logic capabilities of AI, these two advantages will bring the best result. The use of AI technology helps us become more competitive.
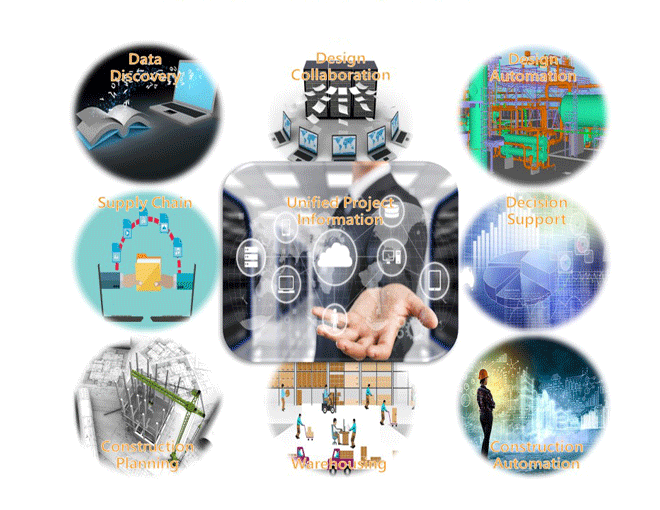
IEPC 8 + 1 Digital Initiatives Road Map
Summary
Group Chairman John T. Yu has said that CTCI's ability in producing sustainable innovation is an important factor that keeps competitors from getting ahead. Along with the advancement of technology, CTCI will continue to introduce various technology in each stage of EPCK. AI combined with image recognition technology for various areas of engineering application shall be studied, such as using video data tags to mark huge data of on-site images autonomously. The video data tagged can be quickly classified in an autonomous fashion, so that these images can be searched in the future and linked to the engineering software for the analysis of equipment degradation or building damages, or for the autonomous review of internal joints in the equipment, etc. In the foreseeable future, the combination of AI, image recognition technology and engineering-related knowledge will enable a new generation of information systems to be applicable in the engineering industry. In conclusion, the sky is the limit.